The Deep Learning Market size was estimated at USD 7.24 billion in 2024 and expected to reach USD 9.49 billion in 2025, at a CAGR 30.52% to reach USD 35.82 billion by 2030.

Setting the Stage for Deep Learning in the Modern Enterprise
Deep learning has transcended its origins in research laboratories to become a critical driver of innovation across industries. Breakthroughs in neural network architectures and the proliferation of open-source frameworks have fueled unprecedented capabilities in image recognition, natural language understanding, and predictive analytics. Organizations now recognize deep learning not simply as a niche technical pursuit but as a strategic asset capable of delivering improved operational efficiency, enhanced customer experiences, and new revenue streams.
Against this backdrop, this executive summary distills the key dynamics shaping the deep learning landscape. It explores the transformative shifts underpinning next-generation AI solutions, examines the ripple effects of recent policy changes, and delineates the multifaceted market segmentation that informs strategic decision-making. By integrating insights on regional nuances, corporate innovation strategies, and actionable recommendations, it offers decision-makers a concise yet comprehensive view of the challenges and opportunities ahead.
Together, these insights lay the foundation for a deeper exploration of the evolving market, beginning with the technological and structural shifts redefining the competitive field.
From Monolithic Systems to Scalable, Democratized AI Frameworks
Over the past several years, the deep learning ecosystem has undergone a structural metamorphosis as organizations transition from siloed on-premise deployments to hybrid configurations that balance cloud scalability with localized processing. This hybrid paradigm empowers enterprises to leverage elastic compute resources for large-scale training while maintaining data sovereignty and low-latency inference at the edge. Such architectural flexibility has become essential for applications ranging from autonomous systems to real-time analytics in critical infrastructure.
In parallel, the proliferation of specialized hardware accelerators has redefined performance benchmarks. Companies now integrate ASIC-based inference engines alongside GPU clusters and FPGA arrays to optimize energy efficiency and throughput. This heterogeneous approach enables more granular resource allocation and cost control, particularly as workload characteristics diversify across training, development, and inference stages.
Equally significant is the evolution of open-source deep learning frameworks and complementary development tools. These innovations have democratized access to sophisticated neural architectures, fostering a vibrant community of contributors who refine models, share best practices, and drive interoperability across platforms.
Consequently, these converging trends are forging a more agile, collaborative, and cost-effective landscape for deep learning adoption, setting the stage for the subsequent analysis of policy and market segmentation impacts.
Assessing the Ripple Effects of US Tariffs on AI Supply Chains 2025
In 2025, the implementation of new United States tariffs targeting semiconductor imports has introduced a pivotal variable in the deep learning value chain. By levying higher duties on key components such as tensor processors, GPU modules, and advanced ASICs, policymakers aim to recalibrate the balance between domestic production and international supply. While the strategic intent emphasizes bolstering onshore manufacturing, the immediate consequence has been an uptick in input costs for laptop-to-datacenter scale systems.
Hardware vendors, confronted with elevated import expenses, have recalibrated pricing models for both training clusters and inference appliances. These adjustments ripple across research institutions and enterprise buyers, prompting procurement teams to reassess total cost of ownership. Meanwhile, downstream integrators have begun to explore alternative supply partners and negotiate longer-term contractual commitments to mitigate volatility.
In response, a growing cohort of manufacturers and distributors have invested in regional assembly hubs and diversified component sourcing. This strategic pivot aims to localize critical stages of the hardware value chain, reduce cross-border dependencies, and insulate end users from abrupt policy shifts.
As a result, software and firmware optimization has gained renewed prominence. Development teams are prioritizing quantization techniques and model compression to stretch hardware investments further, ensuring that performance gains remain attainable despite tariff-induced budget constraints.
Unpacking Market Dimensions: Deployment, Components, Industries, and Applications
A critical lens on deployment reveals two dominant modalities in the deep learning market: cloud-based environments and on-premise infrastructures. Cloud platforms continue to attract organizations seeking elastic compute capacity for large-scale model training, while on-premise solutions find favor in sectors demanding stringent data privacy and real-time inference capabilities. Adaptive hybrid deployments are increasingly prevalent, offering orchestration across both realms.
The component architecture further stratifies the ecosystem. On the hardware front, specialized ASICs, GPUs, CPUs, and FPGAs each deliver unique performance and power-efficiency trade-offs, enabling tailored configurations for training and inference workloads. Professional services and managed service offerings complement hardware portfolios, providing system integration, optimization, and ongoing support. Software layers encompassing deep learning frameworks, developer toolkits, and inference engines facilitate model development, experimentation, and deployment, driving productivity and interoperability.
Industry vertical segmentation underscores how deep learning applications influence distinct sectors. Automotive firms apply neural networks to autonomous navigation, while financial institutions utilize predictive analytics for risk management. Healthcare providers leverage medical imaging and diagnostics, and retail enterprises implement personalized recommendations. Government agencies and defense organizations prioritize surveillance and threat detection, whereas telecommunications operators integrate network optimization solutions. Manufacturing facilities adopt predictive maintenance protocols to reduce downtime.
Organizational scale also shapes adoption strategies. Large enterprises typically incorporate extensive AI centers of excellence and invest in end-to-end deployments, whereas small and medium businesses often pursue targeted proofs of concept or leverage managed services to accelerate time to value.
Diverse application domains span autonomous vehicles, advanced image recognition for facial identification and object classification, natural language processing solutions like chatbots and sentiment analysis, as well as speech recognition services. These use cases highlight the broad spectrum of neural architectures and inference pipelines that organizations tailor to specific business needs.
This comprehensive research report categorizes the Deep Learning market into clearly defined segments, providing a detailed analysis of emerging trends and precise revenue forecasts to support strategic decision-making.
- Deployment Mode
- Component
- Industry Vertical
- Organization Size
- Application
Geographical Dynamics Shaping Deep Learning Adoption Globally
In the Americas, expansive cloud infrastructure and robust venture capital ecosystems have accelerated deep learning adoption across both mature and emerging industries. North American research institutions continue to pioneer novel neural architectures, while enterprises in sectors such as automotive and finance integrate AI-driven solutions into their core operations. Latin American markets, though at earlier stages of maturity, exhibit growing demand for scalable analytics platforms and cloud-based services that address local challenges in agriculture, energy, and e-commerce.
Across Europe, the Middle East, and Africa, regulatory considerations and data sovereignty requirements exert significant influence on deployment choices. European Union directives on AI ethics and data protection encourage the development of privacy-preserving machine learning techniques and drive investment in federated learning initiatives. Meanwhile, Middle Eastern governments fund ambitious smart city and defense programs, and African startups leverage mobile-based inference solutions to deliver financial inclusion and healthcare accessibility in areas with limited infrastructure.
In the Asia-Pacific region, aggressive digital transformation strategies and substantial public and private sector investment have propelled rapid deep learning integration. Leading technology hubs in East Asia underpin global supply chains for advanced semiconductors, while Southeast Asian nations adopt AI-powered logistics, retail, and telemedicine platforms. Collaboration between academic institutions and industry players fosters an innovation ecosystem that continuously refines algorithms and adapts them to regional linguistic, environmental, and cultural contexts.
This comprehensive research report examines key regions that drive the evolution of the Deep Learning market, offering deep insights into regional trends, growth factors, and industry developments that are influencing market performance.
- Americas
- Europe, Middle East & Africa
- Asia-Pacific
Leading Pioneers and Emerging Contenders in the Deep Learning Arena
The competitive landscape for deep learning is defined by a mix of established technology providers, emerging startups, and collaborative alliances that drive innovation and commercialization. Leading hardware vendors continue to refine their chip architectures, balancing compute density with energy efficiency to support increasingly complex neural networks. Meanwhile, cloud infrastructure giants invest heavily in specialized AI accelerators and optimized data pipelines, ensuring that enterprises can access high-performance training and inference environments on demand.
On the software side, open-source framework maintainers and proprietary tool developers shape the ease of model creation and deployment. Continuous enhancements in development environments, model interpretability libraries, and automated machine learning platforms empower data science teams to iterate more rapidly and reduce time to deployment. Several key players have also established partner ecosystems that integrate third-party modules, enhancing model versatility across industry-specific use cases.
Strategic alliances between hardware suppliers and system integrators have emerged as a critical enabler for large-scale implementations. By co-designing solutions that span custom silicon, firmware, and software stacks, these partnerships address performance bottlenecks and streamline deployment workflows, particularly for clients requiring low-latency or resource-constrained operations.
At the forefront of innovation, newer entrants specializing in niche applications-such as edge inference optimization and privacy-preserving AI-are carving out market share by addressing specific pain points. Their agility and domain expertise complement the broader portfolios of established incumbents, resulting in a dynamic competitive environment where collaboration and specialization coexist.
This comprehensive research report delivers an in-depth overview of the principal market players in the Deep Learning market, evaluating their market share, strategic initiatives, and competitive positioning to illuminate the factors shaping the competitive landscape.
- NVIDIA Corporation
- Microsoft Corporation
- Alphabet Inc.
- Amazon.com, Inc.
- International Business Machines Corporation
- Meta Platforms, Inc.
- Intel Corporation
- Baidu, Inc.
- Huawei Technologies Co., Ltd.
- Tencent Holdings Limited
Strategic Imperatives for Sustained Competitive Advantage
To maintain a sustainable competitive edge, industry leaders must prioritize investments in adaptive infrastructures that encompass both cloud and edge environments. This dual approach ensures that organizations can scale compute resources for intensive training tasks while delivering low-latency inference in mission-critical scenarios. By leveraging container orchestration and automated deployment pipelines, teams can optimize resource utilization and accelerate time to market.
In parallel, diversifying the supply chain for critical hardware components mitigates disruption risks associated with policy shifts or geopolitical tensions. Establishing strategic partnerships with regional assembly hubs and component manufacturers enhances resilience and can unlock cost efficiencies through longer-term procurement agreements.
Developing internal deep learning expertise remains essential. Organizations should expand talent pipelines by offering specialized training programs and collaborating with academic institutions to foster practical skills in neural network design, model optimization, and interpretability techniques. Encouraging cross-functional collaboration between data scientists, IT operations, and business units also promotes alignment on strategic objectives.
Finally, implementing robust AI governance frameworks-addressing model transparency, ethical considerations, and data privacy-will build stakeholder trust and ensure compliance with evolving regulatory landscapes. Integrating continuous monitoring and feedback mechanisms into production systems enables ongoing performance tuning and risk management.
Rigorous Methodology Underpinning Insights and Analysis
This report’s insights derive from a rigorous methodological framework combining primary interviews with industry executives, technical experts, and end users across core sectors. These conversations provided nuanced perspectives on deployment strategies, cost dynamics, and emerging pain points. Complementing these qualitative inputs, a comprehensive review of secondary sources-including white papers, regulatory filings, and peer-reviewed publications-ensured a holistic understanding of both technological trends and policy developments.
Data triangulation played a pivotal role in validating findings. Market intelligence from proprietary databases on supply chain movements and patent activity was cross-referenced with real-time telemetry from selected development platforms. This layered approach offered both breadth and depth, revealing correlations between hardware innovations, software advancements, and adoption trajectories in varied geographical contexts.
To enhance analytical rigor, quantitative metrics were supplemented with case study analyses of representative deployments. Performance benchmarks, total cost considerations, and time-to-value assessments informed a series of comparative evaluations that underpin the strategic recommendations. Ethics and data privacy considerations were also factored into the methodology to reflect the governance imperatives shaping solution design and procurement decisions.
Explore AI-driven insights for the Deep Learning market with ResearchAI on our online platform, providing deeper, data-backed market analysis.
Ask ResearchAI anything
World's First Innovative Al for Market Research
Synthesizing Key Takeaways and Forward Pathways
The synthesis of technological, policy, and market segmentation analyses underscores a deep learning landscape characterized by both rapid innovation and heightened complexity. Hybrid deployment models and specialized hardware accelerators have redefined performance parameters, while open-source ecosystems continue to democratize access to cutting-edge tools. However, emerging trade policies, particularly the 2025 tariff adjustments, have introduced new supply chain challenges that require strategic mitigation.
Regional dynamics further complicate the global picture, with variations in regulatory frameworks, infrastructure maturity, and investment climates shaping localized adoption patterns. Leading companies and agile startups alike must navigate this heterogeneous environment by aligning product roadmaps to regional priorities and forging collaborative partnerships that harness complementary strengths.
Ultimately, organizations that integrate robust governance structures, diversify sourcing strategies, and cultivate deep learning expertise will be best positioned to capitalize on next-generation AI capabilities. The insights and recommendations presented herein offer a pathway for decision-makers to navigate uncertainty, optimize resource allocation, and drive sustainable competitive advantage in this dynamic market.
This section provides a structured overview of the report, outlining key chapters and topics covered for easy reference in our Deep Learning market comprehensive research report.
- Preface
- Research Methodology
- Executive Summary
- Market Overview
- Market Dynamics
- Market Insights
- Cumulative Impact of United States Tariffs 2025
- Deep Learning Market, by Deployment Mode
- Deep Learning Market, by Component
- Deep Learning Market, by Industry Vertical
- Deep Learning Market, by Organization Size
- Deep Learning Market, by Application
- Americas Deep Learning Market
- Europe, Middle East & Africa Deep Learning Market
- Asia-Pacific Deep Learning Market
- Competitive Landscape
- ResearchAI
- ResearchStatistics
- ResearchContacts
- ResearchArticles
- Appendix
- List of Figures [Total: 26]
- List of Tables [Total: 465 ]
Partner with Ketan Rohom to Access Full Deep Learning Market Analysis
For personalized guidance on leveraging these insights, connect with Ketan Rohom, Associate Director of Sales & Marketing. His expertise in deep learning market dynamics can help you identify the precise research deliverables and custom analyses that align with your strategic priorities.
Secure immediate access to the full market research report to inform your next steps in developing scalable AI initiatives, optimizing your hardware and software investments, and navigating regulatory complexities. Reach out today to explore tailored solutions that will empower your organization to lead in the era of intelligent automation.
Engage now to transform data-driven insights into actionable plans that accelerate innovation and drive long-term growth
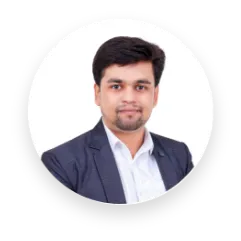
- How big is the Deep Learning Market?
- What is the Deep Learning Market growth?
- When do I get the report?
- In what format does this report get delivered to me?
- How long has 360iResearch been around?
- What if I have a question about your reports?
- Can I share this report with my team?
- Can I use your research in my presentation?