The Federated Learning Solutions Market size was estimated at USD 166.34 million in 2024 and expected to reach USD 192.71 million in 2025, at a CAGR 15.27% to reach USD 390.36 million by 2030.
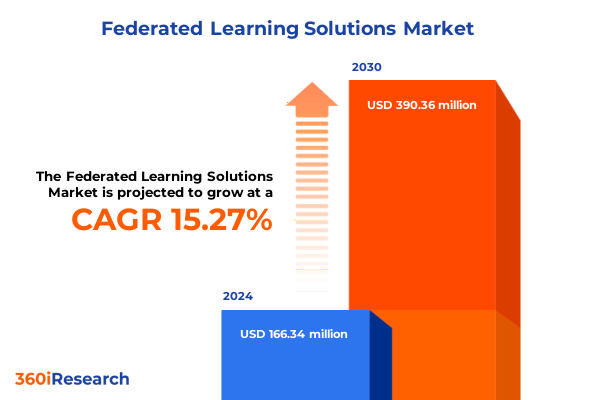
Introduction to Federated Learning Solutions
Federated learning has emerged as a transformative paradigm in which multiple organizations or devices jointly train machine learning models without sharing sensitive local data. By orchestrating model updates rather than raw datasets, this approach mitigates privacy risks, aligns with evolving data regulations, and reduces communication overhead through decentralized training. At its core, federated learning leverages advancements in edge computing and secure aggregation to enable intelligent insights across heterogeneous environments. Key stakeholders-including automotive manufacturers, financial institutions, healthcare providers, and technology vendors-are exploring federated frameworks to unlock collaborative analytics, optimize operational efficiency, and maintain compliance with stringent data protection standards. As the competitive landscape pivots toward data-driven innovation, federated learning solutions offer a compelling path to harness distributed intelligence while safeguarding individual privacy and organizational confidentiality.
Transformative Shifts in the Federated Learning Landscape
The landscape of federated learning is undergoing rapid evolution, driven by technological breakthroughs, regulatory pressures, and heightened expectations for data privacy. Advances in model architectures and communication protocols have accelerated convergence rates and reduced latency in edge deployments. Simultaneously, emerging standards for secure multiparty computation and differential privacy are fostering greater trust among participants in collaborative frameworks. Regulatory developments-such as updates to GDPR, HIPAA, and state-level privacy laws-are compelling enterprises to adopt architectures that minimize data exposure. At the same time, the proliferation of IoT devices and 5G networks has expanded the horizon for real-time learning at the edge, enabling applications from autonomous vehicles to smart manufacturing. These shifts are fundamentally reorienting how organizations approach data sharing, collaboration, and model governance, creating opportunities for new entrants and strategic partnerships among incumbent technology providers and domain specialists.
Cumulative Impact of United States Tariffs in 2025
The imposition of tariffs on key hardware components and communication infrastructure in 2025 has introduced significant cost pressures across the federated learning ecosystem. Tariffs on advanced semiconductors, networking equipment, and sensor modules have driven up expenses for data centers and edge devices alike, prompting organizations to reexamine procurement strategies. In response, many stakeholders are shifting toward diversified supplier networks and investing in domestically manufactured hardware to mitigate supply chain disruptions. Moreover, increased duties on cloud infrastructure imports have accelerated the adoption of hybrid deployment models that emphasize local processing and data residency. While these measures have temporarily dampened investment in large-scale federated deployments, they have also catalyzed innovation in hardware-agnostic software frameworks and open-source toolchains designed to optimize resource utilization under constrained budgets. Overall, the tariff environment of 2025 has underscored the need for resilient supply chains and flexible deployment architectures in sustaining federated learning initiatives.
Key Segmentation Insights
Analysis of federated learning solutions across application, technology, end-user, solution type, and deployment segments reveals distinct patterns in adoption and innovation. In application areas, autonomous vehicles lead with use cases such as driver behavior analysis, object detection, and traffic prediction, while education platforms leverage curriculum development, performance analytics, and personalized learning to enhance student outcomes. Financial institutions focus on credit scoring, fraud detection, and risk management, and healthcare organizations prioritize drug development, medical imaging analysis, and patient data management. Meanwhile, the retail sector invests in in-store customer experience optimization, inventory management, and personalized recommendations. Technological components span hardware, network infrastructure, and software: data centers, edge, and mobile devices are driving compute acceleration, communication protocols and security solutions are fortifying distributed networks, and sophisticated algorithms, collaborative platforms, and data processing tools are refining model training and aggregation. From an end-user perspective, large enterprises emphasize cross-department analytics, scalability concerns, and strategic implementation, whereas small and medium enterprises navigate challenges around investment outlook, solutions adoption rate, and operational flexibility. When selecting solution types, organizations often choose managed services-encompassing consultancy, deployment, and maintenance-and professional services, which deliver audit and optimization, system integration, and training. Deployment models are equally bifurcated: cloud-based architectures, including hybrid, private, and public cloud offerings, provide elastic scalability, and on-premises implementations require careful infrastructure planning, resource allocation, and security protocols to meet stringent compliance mandates.
This comprehensive research report categorizes the Federated Learning Solutions market into clearly defined segments, providing a detailed analysis of emerging trends and precise revenue forecasts to support strategic decision-making.
- Application Areas
- Technological Components
- End-User Verticals
- Solution Type
- Deployment Model
Regional Dynamics and Adoption Patterns
The federated learning market exhibits region-specific dynamics that reflect variations in regulatory frameworks, technological maturity, and industry priorities. In the Americas, North American enterprises benefit from robust investment in edge computing and strong collaboration between technology firms and academic research institutions, while Latin American organizations are increasingly adopting federated models to address data sovereignty and emerging privacy regulations. Across Europe, Middle East, and Africa, GDPR compliance and cross-border data flow restrictions are shaping federated deployments, with Western Europe leading in collaborative healthcare and smart city projects, and entities in the Middle East and Africa pursuing pilot programs that demonstrate proof of concept in finance and energy. In the Asia-Pacific region, accelerated by government initiatives and 5G rollouts, federated learning is gaining traction in manufacturing, telecommunications, and autonomous transportation, with China and Japan spearheading large-scale industrial applications and India emphasizing cost-effective, scalable solutions for educational and financial services.
This comprehensive research report examines key regions that drive the evolution of the Federated Learning Solutions market, offering deep insights into regional trends, growth factors, and industry developments that are influencing market performance.
- Americas
- Asia-Pacific
- Europe, Middle East & Africa
Competitive Landscape and Company Highlights
The competitive landscape is populated by a diverse array of stakeholders spanning startups, cloud providers, hardware vendors, and research-focused enterprises. Innovative newcomers like Acuratio Inc., apheris AI GmbH, and Everest Global, Inc. are differentiating through specialized privacy-preserving algorithms and niche vertical deployments. Established technology giants-Google LLC, Microsoft Corporation, and International Business Machines Corporation-offer comprehensive federated learning platforms integrated with their cloud and AI ecosystems. Semiconductor leaders such as Intel Corporation and Nvidia Corporation are delivering optimized hardware for edge training, while Hewlett Packard Enterprise Development LP and Oracle Corporation extend federated capabilities through enterprise-grade infrastructure solutions. Domain-specific players, including Lifebit Biotech Ltd. and Owkin Inc., focus on biomedical research and drug discovery use cases, whereas Cloudera, Inc. and SAP SE cater to enterprise data management and analytics. Additional contributors like BranchKey B.V., Duality Technologies Inc., Enveil, Inc., Faculty Science Limited, FedML, Integral and Open Systems, Inc., LiveRamp Holdings, Inc., Secure AI Labs, Sherpa Europe S.L., SoulPage IT Solutions, TripleBlind, WeBank Co., Ltd., Zoho Corporation Pvt. Ltd., Aptima, Inc., BranchKey B.V., Consilient, Edge Delta, Inc., Ekkono Solutions AB, Integral and Open Systems, Inc., and TripleBlind are carving out market share through differentiated service offerings, from edge-native orchestration to advanced cryptographic techniques and sector-specific consultancy.
This comprehensive research report delivers an in-depth overview of the principal market players in the Federated Learning Solutions market, evaluating their market share, strategic initiatives, and competitive positioning to illuminate the factors shaping the competitive landscape.
- Acuratio Inc.
- apheris AI GmbH
- Aptima, Inc.
- BranchKey B.V.
- Cloudera, Inc.
- Consilient
- Duality Technologies Inc.
- Edge Delta, Inc.
- Ekkono Solutions AB
- Enveil, Inc.
- Everest Global, Inc.
- Faculty Science Limited
- FedML
- Google LLC by Alphabet Inc.
- Hewlett Packard Enterprise Development LP
- Integral and Open Systems, Inc.
- Intel Corporation
- Intellegens Limited
- International Business Machines Corporation
- Lifebit Biotech Ltd.
- LiveRamp Holdings, Inc.
- Microsoft Corporation
- Nvidia Corporation
- Oracle Corporation
- Owkin Inc.
- SAP SE
- Secure AI Labs
- Sherpa Europe S.L.
- SoulPage IT Solutions
- TripleBlind
- WeBank Co., Ltd.
- Zoho Corporation Pvt. Ltd.
Actionable Strategies for Accelerating Adoption
To navigate the evolving federated learning landscape, industry leaders should prioritize a multilayered strategy. First, invest in modular, hardware-agnostic software frameworks that can adapt to tariff-induced supply chain volatility and maintain performance across heterogeneous devices. Second, establish strategic alliances with regional research institutions and open-source consortia to accelerate innovation in privacy-preserving algorithms and secure aggregation protocols. Third, implement governance models that align with global and local regulations, integrating automated compliance checks and audit trails to build stakeholder trust. Fourth, pilot hybrid deployment architectures that balance cloud-based elasticity with on-premises control, optimizing data residency and latency requirements. Finally, develop tailored go-to-market strategies that address the unique needs of large enterprises and small and medium enterprises, offering flexible service bundles-ranging from managed deployments to professional audit and integration services-to maximize adoption and long-term customer value.
Explore AI-driven insights for the Federated Learning Solutions market with ResearchAI on our online platform, providing deeper, data-backed market analysis.
Ask ResearchAI anything
World's First Innovative Al for Market Research
Conclusion and Future Outlook
Federated learning solutions are redefining the boundaries of collaborative AI by providing a scalable, privacy-centric framework for distributed model training. Driven by advances in edge computing, secure communication protocols, and supportive regulatory trends, federated architectures are enabling transformative applications across industries-from autonomous driving and precision medicine to smart retail and financial risk mitigation. While challenges remain, including supply chain uncertainties and evolving compliance landscapes, strategic investments in adaptable technology stacks and robust governance frameworks will pave the way for sustainable growth. Organizations that embrace this approach today stand to gain a competitive edge through enhanced data insights, heightened trust, and optimized resource efficiency.
This section provides a structured overview of the report, outlining key chapters and topics covered for easy reference in our Federated Learning Solutions market comprehensive research report.
- Preface
- Research Methodology
- Executive Summary
- Market Overview
- Market Dynamics
- Market Insights
- Cumulative Impact of United States Tariffs 2025
- Federated Learning Solutions Market, by Application Areas
- Federated Learning Solutions Market, by Technological Components
- Federated Learning Solutions Market, by End-User Verticals
- Federated Learning Solutions Market, by Solution Type
- Federated Learning Solutions Market, by Deployment Model
- Americas Federated Learning Solutions Market
- Asia-Pacific Federated Learning Solutions Market
- Europe, Middle East & Africa Federated Learning Solutions Market
- Competitive Landscape
- ResearchAI
- ResearchStatistics
- ResearchContacts
- ResearchArticles
- Appendix
- List of Figures [Total: 26]
- List of Tables [Total: 865 ]
Secure the Comprehensive Federated Learning Report Today
To access an in-depth analysis and strategic guidance on federated learning solutions, contact Ketan Rohom (Associate Director, Sales & Marketing) to secure your copy of the comprehensive market research report.
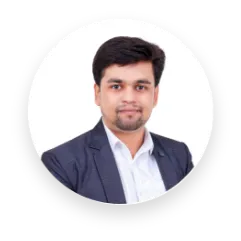
- How big is the Federated Learning Solutions Market?
- What is the Federated Learning Solutions Market growth?
- When do I get the report?
- In what format does this report get delivered to me?
- How long has 360iResearch been around?
- What if I have a question about your reports?
- Can I share this report with my team?
- Can I use your research in my presentation?